ElasticSearch, a powerful and versatile search and analytics engine, has emerged as a cornerstone technology for managing and querying large-scale data with unprecedented speed and precision. However, as the size and complexity of data repositories continue to grow, so do the challenges associated with retrieving the most relevant results.
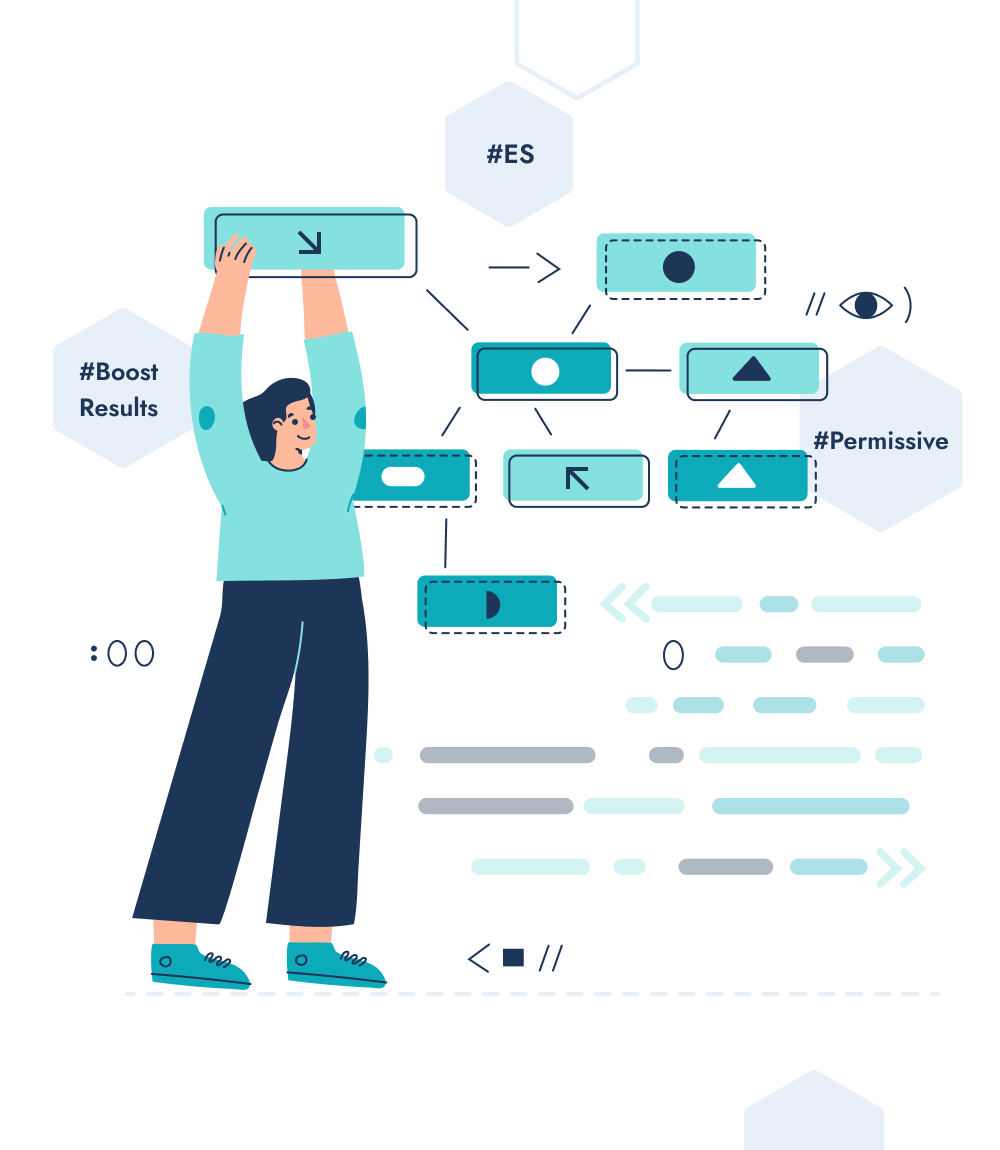
How to deal with it? You can easily optimize search outcomes by using permissive and strict analyzers to fine-tune result filtering and boosting.
The Difference Between Permissive and Strict Analyzers
Using a boolean query we can take different occurrence types. Some of them are:
- must – The clause (query) must appear in matching documents and will contribute to the score.
- should – The clause (query) should appear in the matching document.
Permissive query rules used in “must” can be treated like a filter that will be responsible for picking matched documents from index. It aims to broaden the scope of search results by accommodating variations in spelling, stemming, and synonyms, ensuring that users encounter a comprehensive array of relevant documents.
Strict query rules used in “should” can be treated like some kind of booster that will push documents higher in the relevancy position because matching documents will get a higher score.
How to Use it?
Let’s start from preparing permissive and strict analyzers:
“permissive": { "char_filter": [ "html_strip" ], "tokenizer": "icu_tokenizer", "filter": [ "english_possessive_stemmer", "english_stemmer", "custom_length" ] }
And now strict analyzer:
"strict": { "char_filter": [ "html_strip" ], "tokenizer": "icu_tokenizer", "filter": [ "english_possessive_stemmer", "custom_length" ] }
To keep examples as small as possible please note only one difference between permissive and strict analyzer is one additional filter “english_stemmer”.
Now let’s continue to index structure definition. In order to keep different analyzed contents inside the index we will use “fields” feature.
"name": { "type": "text", "analyzer": "permissive", "fields": { "strict": { "type": "text", "analyzer": "strict" } } }, "description": { "type": "text", "analyzer": "permissive", "fields": { "strict": { "type": "text", "analyzer": "strict" } } },
And finally let’s go build our query that will use “must”, “should” and different analyzers to filter out and boost results.
"query": { "bool": { "must": [ { "multi_match": { "query": "swimming", "fields": [ "name", "description" ], "analyzer": "permissive", } ], "should": [ { "multi_match": { "query": "swimming", "fields": [ "name.strict", "description.strict" ], "analyzer": "strict", } } ] } }
In the example all documents that contain any kind of “swim” form of verb like “swimming”, “swim”, “swam” will be picked up by “must” rules because of permissive analyzer keep “english_stemmer” filter that will reduce english verbs to their root form. Using the same analyzer in query time will always reduce input term to root form.
On the other hand, a strict analyzer that does not have an “english_stemmer” filter will search documents that have exactly the same form as the customer is trying to search. But as a strict analyzer is used in the “should” part of the query it will not filter out products that do not match. It will give higher scores to products that match terms in the same form.
To give more ideas how to use permissive and strict methods please keep in mind that any of the available filters can be used to build different analyzers.
Good to Remember
- Synonyms for example is also a good filter that works very well with permissive and strict analyzers.
- On top of that please remember “should” can have multiple query rules. Each of the query rules can use a different strict analyzer.
- Method is very flexible and very efficient in order to provide better search experience and better results to the customer.