In recent years, the integration of artificial intelligence has surged across industries, transforming various operations from healthcare to finance. However, this rapid advancement has also raised significant ethical concerns.
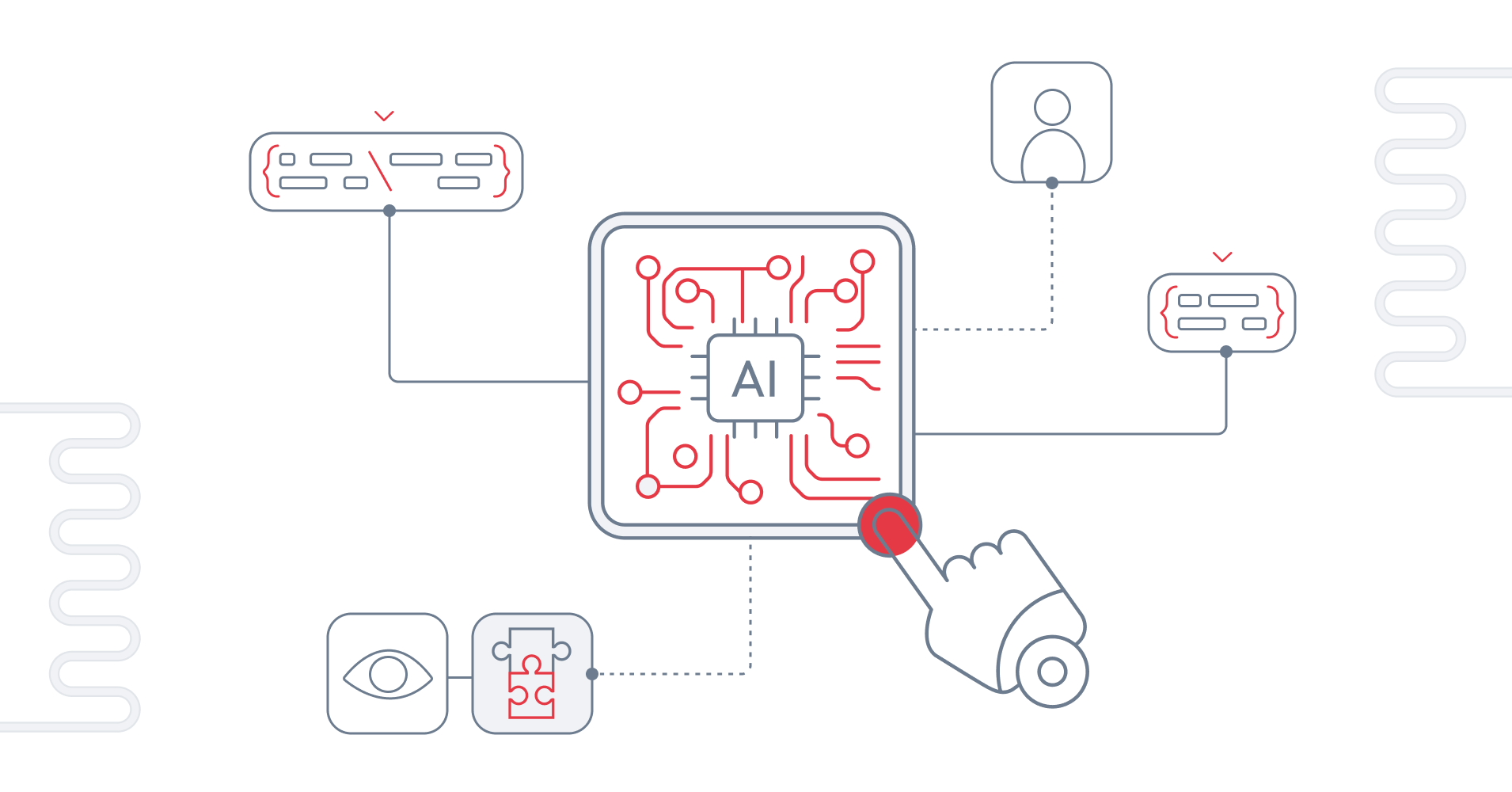
Only 2% of companies declare that they have implemented responsible AI across their organization, although 31% expect to do it in the next 18 months. Major industry leaders like Accenture or IBM have already incorporated responsible AI practices into their operations to reduce risks and gain trust.
Understanding and addressing these ethical issues is critical for ensuring the beneficial use of AI. Today, we take a closer look at the best practices for ethical AI implementation to help you navigate this complex topic.
What Is Ethical AI and Why Is It Important?
Ethical AI refers to the design, development, and deployment of AI systems in ways that are fair, transparent, and accountable. It aims to ensure that AI technologies operate in a manner that is aligned with societal values and ethical principles.
Implementing ethical AI is crucial for several reasons. It helps build trust with users and stakeholders by demonstrating a commitment to responsible AI use. Ethical AI also mitigates risks related to bias, discrimination, and privacy violations, which can have severe legal and reputational consequences. Moreover, ethical AI fosters innovation by encouraging the development of systems that are not only effective but also just and equitable.
Establishing an Ethical Framework
To implement ethical AI, organizations should start by defining clear ethical principles. These principles often include fairness (ensuring AI does not discriminate), accountability (establishing responsibility for AI outcomes), and transparency (making AI decisions understandable). Other principles might include respect for privacy, security, and promoting human well-being.
Above that, keeping abreast of global AI regulations and standards is essential. Regulations such as the European Union's GDPR and AI Act set strict requirements for data protection and ethical AI usage. Organizations must ensure their AI practices comply with these regulations to avoid legal penalties and maintain public trust.
Transparency Is the Key
Transparency in AI involves making the processes and decisions of AI systems clear and understandable to all stakeholders. This transparency is critical for building trust and allowing users to understand how and why decisions are made.
Explainability goes a step further by ensuring that AI decisions can be interpreted and understood by humans. This is particularly important in high-stakes areas like healthcare and criminal justice, where understanding the rationale behind AI decisions is crucial.
Useful Tools and Techniques
There are several tools and techniques available to enhance AI transparency and explainability. Model-agnostic methods, such as LIME (Local Interpretable Model-agnostic Explanations) and SHAP (SHapley Additive exPlanations), can be used to interpret complex models. Additionally, organizations should document their AI systems' decision-making processes and make this documentation accessible to stakeholders.
Accountability
Establishing a clear accountability framework is essential for ethical AI implementation. This framework should delineate the roles and responsibilities of all individuals and teams involved in the development, deployment, and maintenance of AI systems. By assigning clear accountability, organizations can ensure that ethical considerations are integrated at every stage of the AI lifecycle.
Continuous monitoring and evaluation of AI systems are crucial to maintaining ethical standards and addressing emerging issues. Organizations should implement robust monitoring processes to track the performance and impact of AI systems over time.
Bias and Fairness
Bias in AI systems can result from biased training data, which reflects historical inequalities and prejudices. Algorithms may also introduce bias through flawed design or implementation, leading to unfair outcomes that disproportionately affect certain groups.
To detect and reduce bias, organizations should employ diverse datasets that are representative of all relevant groups. Regular bias audits and impact assessments can help identify and address potential biases in AI systems.
In the previous article, we explained how to mitigate AI bias in greater detail.

Audit and Control
Establishing clear reporting mechanisms for ethical concerns and issues is vital for accountability. Employees and stakeholders should have accessible channels to report ethical concerns without fear of retaliation. Effective reporting mechanisms include creating feedback loops to continuously gather input from users and stakeholders on the ethical performance of AI systems and developing procedures for incident reporting and response, ensuring that ethical issues are promptly addressed and resolved.
Conducting an ethical AI audit involves a systematic evaluation of AI systems to ensure they adhere to ethical guidelines and standards. It can include:
Checking alignment with ethical guidelines and ensuring legal compliance
Evaluating model explainability and reviewing documentation quality
Evaluating error handling processes
Collecting feedback from users and stakeholders
Documenting audit findings and providing actionable recommendations
Privacy Protection
Ensuring that user data is protected is not just a regulatory requirement but also a moral obligation that builds trust and credibility. AI systems may be vulnerable to attacks or flaws that may compromise their functioning or output, resulting in harming users. We must ensure that AI systems are secure, resilient, and accountable.
Here are some key aspects and actionable steps to ensure robust privacy protection:
Data minimization – Collect only the data necessary for the AI system to function. Avoid gathering excessive information that is not essential to the core operations of the AI application. This minimizes the risk of data misuse and exposure.
Anonymization – Employ techniques to anonymize personal data, preventing the identification of individuals. This adds an extra layer of protection, ensuring privacy even if data is leaked or accessed without authorization.
Consent management – Ensure that users provide informed consent before their data is collected and used. Clearly explain how data will be used, stored, and shared, and provide users with the option to opt-out if they choose. Transparency in consent processes builds user trust and compliance.
Data encryption – Utilize strong encryption methods for data at rest and in transit to protect sensitive information from unauthorized access and breaches. Encryption acts as a robust defense mechanism against data theft.
Access controls – Implement strict access controls to ensure that only authorized personnel can access personal data. Use authentication and authorization mechanisms to safeguard data, preventing unauthorized access and potential misuse.
Implementing Ethical AI - Common Doubts and Challenge
The journey to achieving ethical AI is fraught with challenges and uncertainties. Let's take a closer look at some of the most common doubts and obstacles faced by organizations striving to implement ethical AI.
Lack of competencies and resources
Developing and maintaining ethical AI systems requires a unique blend of expertise across various domains, including computer science, ethics, law, and social sciences. Many organizations struggle to find professionals who possess this interdisciplinary knowledge.
Moreover, ethical AI initiatives often demand substantial financial and technical resources. Organizations must invest in high-quality data, robust computing infrastructure, and ongoing training for their teams. For smaller companies or those with limited budgets, these requirements can be prohibitively expensive, leading to a gap in the ethical deployment of AI technologies. Improperly implemented ethical AI can damage a company’s reputation or put it at risk of being sued.
Doubts about properly implementing ethical AI
Even with the necessary resources, there are significant doubts about how to properly implement ethical AI. The complexity of AI systems and the rapid pace of advancements can make it difficult to stay abreast of best practices and ethical guidelines.
Companies may struggle with identifying which ethical principles to prioritize and how to embed these into their AI systems effectively. Additionally, the lack of standardized frameworks and guidelines can lead to inconsistent approaches to ethical AI across the industry.
Biased AI leads to incorrect decisions
AI systems are only as good as the data they are trained on. If the data reflects historical or societal biases, the AI will likely perpetuate these biases, leading to unfair and incorrect decisions.
For instance, biased AI can result in discriminatory hiring practices, unfair loan approvals, and biased law enforcement practices. These outcomes can significantly harm individuals and communities, eroding trust in AI technologies. Addressing this challenge requires rigorous data auditing, diverse datasets, and continual monitoring of AI systems to identify and mitigate bias.
We Are the Missing Molecule of Ethical AI Implementation in Your Organization
The complexity of AI systems and the rapid pace of advancements can make it difficult to stay abreast of best practices and ethical guidelines. Our AI experts can help you implement Ethical AI seamlessly and tackle complex challenges with ease. You save your time, money, and stress. Contact us to learn more about what we can do for you.
Implementing ethical AI is undoubtedly challenging, but with concerted effort and a commitment to ethical principles, it is possible to create AI systems that are fair, transparent, and beneficial to all.